Wildfires across California’s diverse landscape: from ignition, spread, to severity
Abstract
Many studies have advanced our knowledge about the dynamics and drivers of total area burned, but much less is known about wildfire ignition probability and fire behavior across the diverse landscape of California. We here use machine learning approaches to model and diagnose the complex interactions between three aspects of wildfires (ignition, fire spread, and burn severity), weather, fuels, local topography, and human activities in the past few decades. Our analysis leverages on explicit fuel and fire behavior information derived from satellite observations.
Our ignition study showed that human ignitions were driven mostly by population and human modification and secondly by vegetation conditions. The probability of lightning caused ignitions, occurring in more remote areas, were controlled by elevation and precipitation events. We derived daily fire spread and area burned since 2012 from MODIS and VIIRS satellite active fire products. The gradient boosting machine learning model explained 75% of variability in daily area burned and 63% of variability in daily rate of spread. We found the relative importance of various drivers varied across sub-ecoregions in controlling fire spread. Fuel characteristics were key determinants for large fires in the Klamath Mountains and Sierra Nevada. Whereas, in the Southern/Baja region, rapid fire spread was predominantly driven by high wind events.
Wildfires has becoming more severe in California’s northern coastal mountains, with high severity burns increasing by 10% per decade. We further examined the fine-scale association between burn severity and a suite of environmental drivers. Drought doubled both total areas burned and the fraction of high severity burns. We found higher temperature further amplified burn severity, as shown by a comparative study between most recent 2012-16 warm drought and previous 1987-1992 cooler drought. The spatial variability of burn severity was a mixed effect of slope, long-term minimum temperature, fuel amount, and fuel moisture; climatic water deficit and short-term weather became dominant factors for fire severity during wetter years.
The machine learning models enables near real-time estimation of ignition probability and risk of rapid spread and high severity. The data-driven results also provide insights on identifying most vulnerable areas and designing localized fire mitigation strategies of land use planning as well as fuel management in a changing climate.
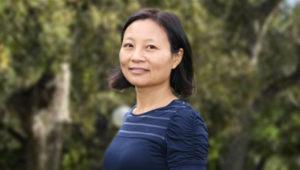
Yufang Jin
Associate Professor
Department of Land, Air, and Water Resources
UC Davis
Biography
Yufang Jin is an associate professor of remote sensing and ecosystem change at the Department of Land, Air, and Water Resources, at University of California, Davis. Her research focuses on satellite remote sensing, ecosystem dynamics, and decision support for sustainable natural and agricultural resource management. She is also interested in machine learning/AI analytics for improved understanding of the interactions between wildfire, vegetation, climate change, and human activities. Dr. Jin received her PhD in Geography from Boston University, and her B.S. degree in Atmospheric Science and her M.S. degree in Environmental Science and Management from Beijing (Peking) University. She worked at UC Irvine and University of Maryland at College Park as a research scientist before joining UC Davis in 2014.